June 8, 2017
Setting Priorities for Quality Improvement (Part 2/4)
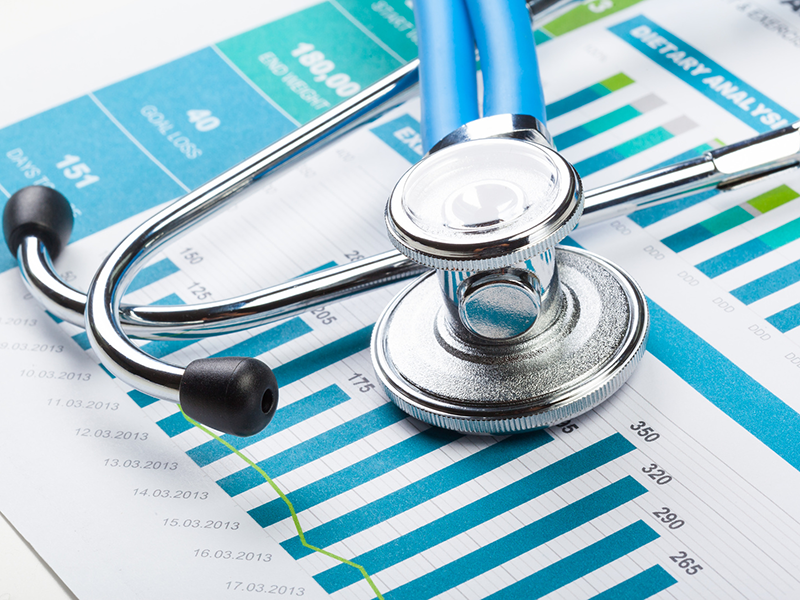
Rigorous, data-driven improvement collaboratives have been shown to accelerate quality improvement and reduce unwanted variation in outcomes and costs. As I’ve described previously, the Northern New England collaborative in cardiovascular care laid out the basic cornerstones of the quality improvement model 25 years ago: effective leadership, engaged clinicians, and a robust clinical registry to provide timely, credible performance feedback and insights on best practice. My experience with the Blue Cross Blue Shield-supported collaboratives in Michigan demonstrated that this model can drive meaningful improvement across almost any specialty and on a much larger scale. Regardless of scale, the mechanics of data-driven quality improvement are straightforward. The first step is picking a high leverage target for improvement, whether a specific patient outcome, clinical practice, and opportunity for cost reduction. A plan for redesigning care is then developed, based on published evidence, analysis of local data, or sometimes clinical intuition and consensus. Third, the intervention is implemented into practice, navigating existing clinical work flows and potential barriers to adoption. And finally, the intervention is evaluated for its impact on targeted outcomes and refined to enhance effectiveness. Writing about quality improvement feels pretty old school, a throwback to the 80s and 90s. Edwards Deming and Total Quality Management. Plan, Do, Study, Act (PDSA) cycles. Define, Measure, Analyze, Improve, and Control (DMAIC) from Six Sigma. These terms are familiar not only to health services researchers and quality leaders, but also most clinicians working in hospitals and health systems. Nonetheless, applying these techniques effectively is harder than it looks. Too many quality improvement initiatives are ultimately one-off projects with inconsistent, non-durable results. In part 1 of this blog series on quality, I described the basic template for successful, data-driven, collaborative improvement—using examples from my work at Dartmouth, Michigan, and now Sound Physicians. I also reviewed the challenges associated with scaling up the model across large numbers of physicians, hospitals and regions. In later blogs, I’ll consider the specific components of successful quality improvement. Here I’ll start with the most basic question: How to select the right improvement target in the first place?
Reactive quality improvement
Selecting the right target is a critical but often overlooked aspect of successful quality improvement. After all, quality improvement is expensive. It involves considerable offline preparation and inevitably lots of meetings along the continuum of planning, testing and implementation, evaluation and maintenance. Because much of the work involves physicians and other clinicians, the time cost is significant. Organizations also have only so much bandwidth and resources to devote to structured quality improvement work. The expected return on a specific project should be considered against its opportunity cost—the value of a competing, potentially more impactful improvement initiative. In health systems, most quality improvement work tends to be reactive—focused on addressing an immediate need. Sometimes that need is accommodating a request from a clinical champion with a particular interest in tackling a specific problem. In most instances, however, it involves putting out a fire. Examples in the quality and safety realm might include a recent cluster of wrong-site surgical procedures in the operating room or citations from a Joint Commission site visit. Increasingly, hospitals and health systems are reacting to the root causes of financial penalties under value-based purchasing programs. For example, in my tenure at Dartmouth-Hitchcock, I remember charging a “SWAT team” to address hospital noise and cleanliness as it was dragging down our HCAHPS composite and contributing to a large penalty from CMS. Focusing exclusively on reactive quality improvement does not effectively prioritize high-impact work. It can often result in chasing isolated safety events, niche clinical targets, or the fleeting interests of individuals or external stakeholders. At the same time, crises always arise and need to be addressed. There is value in supporting clinical champions who are passionate about improving care in your health system, even if some of their interests are low priority. And finally, quality improvement work that focuses initially on a niche problem can sometimes establish a foothold for much broader, higher impact change.
Case study: Overuse of inferior vena cava filters in bariatric surgery Nancy Birkmeyer and I founded the Michigan Bariatric Surgery Collaborative (MBSC) shortly after we arrived at the University of Michigan in 2004. Supported by Blue Cross Blue Shield of Michigan, MBSC was a statewide improvement collaborative, involving essentially all of the approximately 30 hospitals engaged in bariatric (weight loss) surgery at the time. Given concerns about the safety of bariatric surgery in the early 2000s, the overarching goal of the collaborative was to reduce surgical morbidity and mortality. But there was no obvious consensus are where to start. We began to collect process and outcomes on all patients undergoing bariatric procedures across the state. It would be a while before the registry was mature enough to scrutinize specific outcomes, but data regarding one aspect of clinical practice stood out immediately: preoperative placement of inferior vena cava (IVC) filters. An IVC filter is a badminton birdie-shaped device inserted into the IVC, the major abdominal vein returning blood to the heart, via a $10,000 catheter-based procedure. The basic idea of an IVC filter is to trap blood clots traveling from the lower extremities, thus preventing fatal pulmonary embolism, the leading cause of death after bariatric surgery. On examining early MBSC data, variation in IVC filter use across hospitals was striking. Over half of Michigan bariatric programs never used them, while a half dozen (of the busiest) hospitals were inserting IVC filters in over a quarter of their patients. When we brought these data back to the group, the heavy utilizing surgeons defended their practice, pointing to both clinical theory and scattered studies suggesting IVC filter effectiveness in various clinical populations. Subsequent analysis of the MBSC outcomes data told another story, however. Patients getting prophylactic IVC filters before bariatric were not getting better outcomes. In fact, after careful risk adjustment, these patients were experiencing higher rates of both blood clots and death (NJ Birkmeyer et al., Annals of Surgery, 2010). Moreover, many of those deaths were directly attributable to “snafus” with the IVC filter itself. ![]() |
Strategic quality improvement
Strategy-minded hospital or physician leaders tend to set improvement priorities prospectively according to high priority organizational needs. Those needs include meeting the expectations of external stakeholders. Increasingly, hospital leaders must accommodate the priorities of their parent health system. For example, a Chief Medical Officer at one of Sound Physicians’ partner hospitals recently told me, “2017 is the year of patient experience” for his health system. He explained how the health system has refocused their efforts with incentive payments and improvement work in that domain. In other instances, health system leaders are prioritizing improvement that aligns with evolving payment models. Three years ago, for example, Sound Physicians recognized the emergence of episode payment models—and the need to improve patient outcomes after discharge and reduce the total cost of care around acute care episodes. We invested considerable resources in central support and in the field toward improving transitional care, aiming to enhance advance care planning, reduce over-utilization of skilled nursing facilities and rehab centers following discharge, and shorten length of stay in SNFs when clinically appropriate. In addition to meeting organizational needs, strategic quality improvement requires identifying high priority needs for patients. High priority targets tend to involve high prevalence clinical conditions, or high risk, high cost subsets, like the chronically ill. In my experience, examining variation data is another useful tool in identifying ripe improvement targets. The more variable the outcomes, practice, or costs, the more likely improvement.
Case study: Reducing mortality in cardiac surgery For the Northern New England (NNE) Cardiovascular Disease Study Group in 1989, choosing operative mortality as its main improvement priority was a no-brainer. Although rates have come down considerably since, mortality for coronary artery bypass surgery approached 5%. When combined with heart valve procedures, mortality jumped to almost 10%. Still, the burning platform for focusing on mortality was created by variation data – from both Medicare’s publicly available reports and NNE’s own analyses. As I described in an earlier blog, risk-adjusted mortality rates among the 18 surgeons varied five-fold — from 2% to almost 10%. How best to focus improvement efforts was less clear, however. Patients die after cardiac surgery in very different and often very complicated ways. For example, a patient may start with postoperative bleeding requiring a reoperation, then suffer a stroke resulting in a need for a ventilator, followed by pneumonia, sepsis and eventually death. Each one of these clinical events has its own risk factors and a long list of clinical decisions and processes that might be studied and improved to reduce risks. While I was a research fellow, the NNE group embarked on its now seminal “cause of death” study to help it understand variation in provider performance and ultimately distill a long list of improvement possibilities to a shorter, more practical list (O’Connor et al., Ann Thorac Surg, 1998). In that research, a chart abstractor (me!) pulled the medical records of 384 consecutive deaths occurring at the five northern New England hospitals. For each patient, I recorded the occurrence, date and time of every complication occurring between surgery and the patient’s demise. We then convened a panel of cardiac surgeons, all blinded to the identity of the case, to adjudicate the primary cause of death, from among six mutually exclusive categories (there would have been eight, but we wouldn’t let the surgeons include “act of God” and “patient lost the will to live.”). In assigning cause of death, the surgeons were asked to focus on the “seminal complication”—what’s the first thing that went wrong that, if avoided, would have resulted in a surviving patient? ![]() |
Next in this blog series on quality: The mechanics of redesigning clinical process